Abhishek Gupta is the Founder and Principal Researcher at Montreal AI Ethics Institute and Chair of the Green Software Foundation’s Standards Working Group. Click here for the full article.
AI Systems: environmental impact and growing challenges
AI systems require significant computational resources for various tasks such as training, hyperparameter optimization, and inference. They rely on vast amounts of data, often requiring specialized hardware and data center facilities, consuming electricity and contributing to carbon emissions. The substantial carbon footprint raises concerns about environmental sustainability and social justice implications.
As AI models continue to grow in size, the pace of compute consumption is increasing exponentially. For instance, high-compute AI systems have doubled their consumption every 3.4 months, and this rate may even be faster now. Despite the rapid increase in computational requirements, the improvements in performance achieved by these large-scale AI models are only marginal. Moreover, large-scale AI models introduce challenges such as biases, privacy concerns, vulnerability to attacks, and high training costs.
These challenges are compounded by the fact that the models are widely accessible through public application programming interfaces (APIs), limiting the ability to address the problems downstream (for those wondering, making large-scale AI models accessible through public APIs amplifies the risk of widespread bias and errors due to their extensive use, limited user control, and potential privacy breaches, and raises concerns about security vulnerabilities, scalability costs, and ethical challenges across diverse applications and user groups).
Three fundamental issues in the spotlight
Gupta outlines three core challenges embodied in today’s AI paradigm:
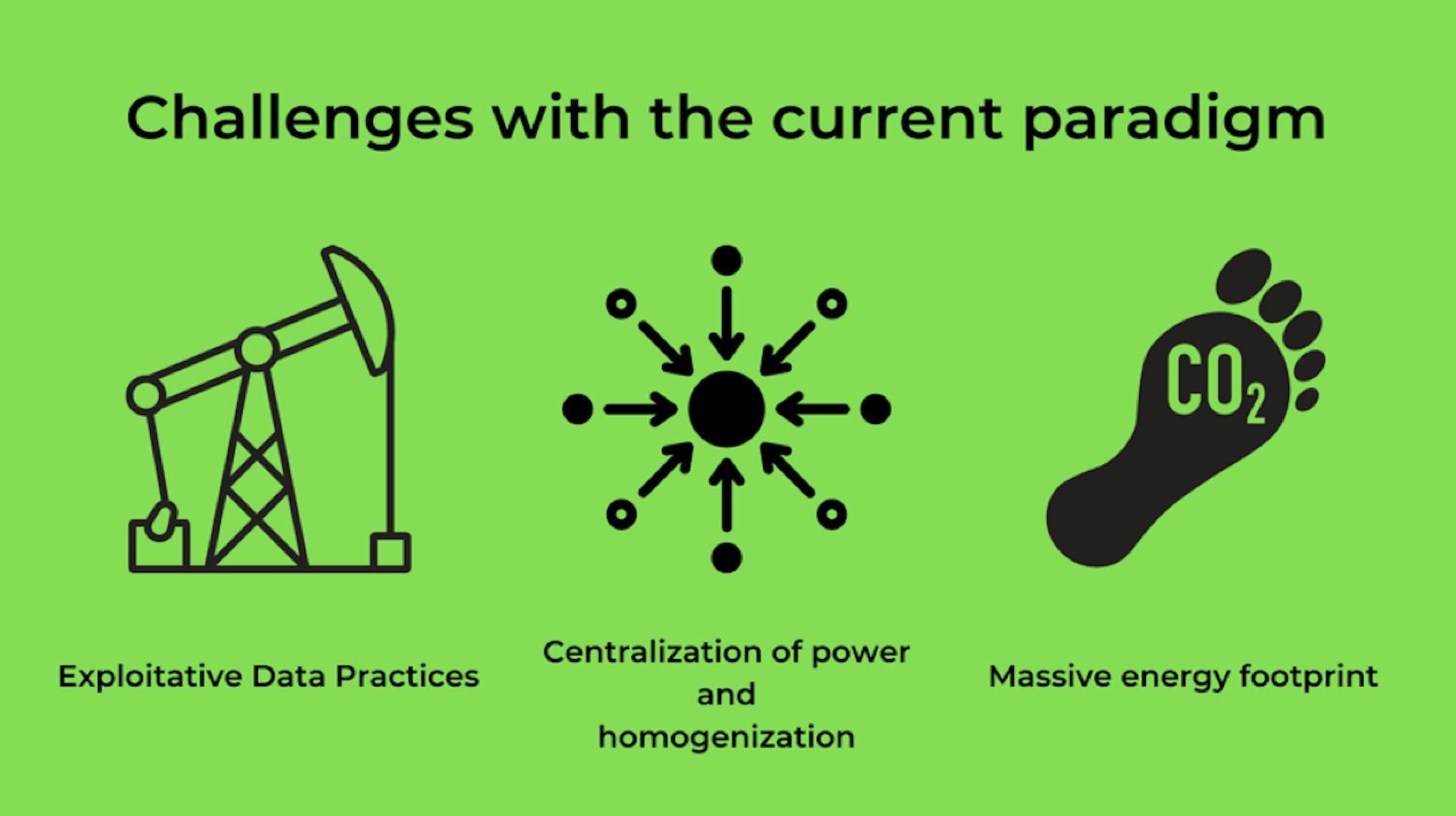
1. Exploitative Data Practice
Large-scale AI models rely on enormous datasets, raising ethical concerns such as lack of informed consent, the inability to retract consent, and challenges in addressing fairness, privacy, and labeling issues. Data often comes from public sources without clear guidelines, allowing for potential misuse and unforeseen consequences, as seen with the repurposing of datasets during the COVID-19 pandemic. Encouraging such exploitative data practices can have significant negative long-term impacts.
2. Massive energy footprints
The environmental impact of AI, notably large models like GPT-3 (and now GPT-4), gained public attention due to concerns about their environmental costs. For instance, a single large NLP model can emit carbon equivalent to five cars in their lifetime. Although standardized methods for measuring these impacts are lacking, various metrics are considered. Assessing AI's environmental effects requires a holistic approach, including hardware and supply chains.
With the urgency of addressing climate change, immediate action is necessary. Data centers now rival the airline industry in emissions, making adopting eco-friendly practices throughout the AI system lifecycle crucial.
3. Centralization of power and homogenization
The AI field already favors specific demographics and regions, with well-funded non-academic organizations wielding significant influence. Those with financial resources can train large models, creating competitive advantages by offering better services and collecting more data. This raises entry barriers for smaller firms lacking data, resources, and operational expertise, solidifying the dominance of incumbents.
In research, well-funded labs enjoy computing resources for extensive searches, faster iterations, and impactful publications, reinforcing their funding prospects. Scarce academic funding and long grant cycles further hinder diversity. Operational costs for cloud resources are prohibitive outside North America and Europe, particularly for addressing societal challenges. To use AI as a force for good, we need to empower local populations to build systems for themselves, ensuring equitable access.
The concept of Sustainable AI
Sustainable AI offers an approach that harmonizes these issues and presents a potential path to addressing them holistically, keeping in mind our planet, organizational profits, and, above all, people at the center of the design, development, and deployment phases of an AI system.
Sustainable AI aims to guide AI system development, focusing on ecological integrity and social justice throughout the AI lifecycle to form a cohesive framework. Gupta suggests incorporating carbon accounting into AI development, gathering relevant data through instrumentation and telemetry, and making carbon impacts a fundamental consideration alongside functional and business requirements. This holistic approach can support the triple bottom line, emphasizing profits, people, and the planet, and contribute to more environmentally and socially responsible AI practices.
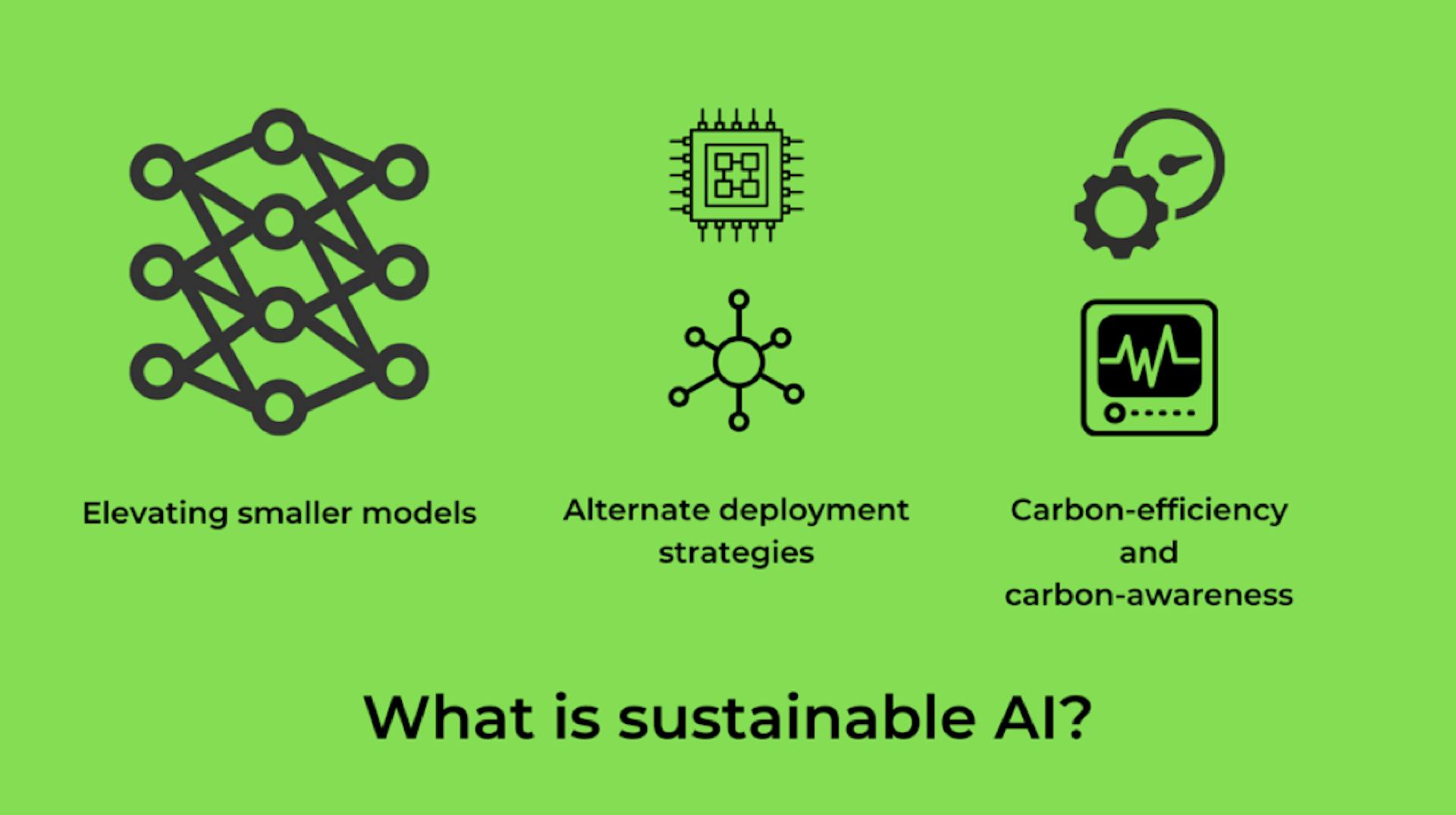
Approaches to solving the challenges of the existing paradigm
Elevating smaller models
Various research efforts are working on training more efficient AI models, employing techniques like pruning, compression, distillation, and quantization. These methods aim to reduce model size and computational demands, ultimately cutting financial and environmental costs.
Additionally, the growth of edge computing and IoT devices with limited resources has led to the emergence of TinyML. Solutions like Bonsai and ProtoNN shrink the model size and prediction costs for resource-restricted devices, while domain-specific languages like SeeDot facilitate AI deployment on edge computing platforms.
Alternate deployment strategies
To reduce the environmental impact of AI systems, three strategies can be employed: (1) using specialized hardware like ASICs and TPUs to accelerate tasks, (2) increasing the utilization of existing hardware to prevent wasteful power consumption, and (3) optimizing the use of general-purpose CPUs to reduce the need for new hardware production. Selecting the proper hardware can yield significant savings in terms of embodied carbon.
Federated learning, a decentralized training technique that keeps data on-device, offers privacy protection and the potential to shift compute-intensive AI tasks to regions with lower carbon intensity, achieving dual objectives with a single change.
Carbon-efficiency and carbon-awareness
Carbon-efficiency means optimizing software and hardware to achieve the desired output with the least computation and energy expenditure. Carbon awareness, on the other hand, involves adjusting an AI system's operational parameters based on the energy grid's carbon intensity. The system's carbon footprint can be reduced by dynamically selecting the most environmentally friendly times and locations for operation.
Energy grid disparities, with up to a 30x difference in carbon intensity, highlight the importance of considering where AI systems are trained and deployed. Tools like the Azure Sustainability Calculator and cloud providers' internal load balancing contribute to achieving sustainability goals in this context.
An appeal to software developers
Moving in the right direction largely depends on the efforts of the software developer community and its willingness to adopt sustainable AI practices.
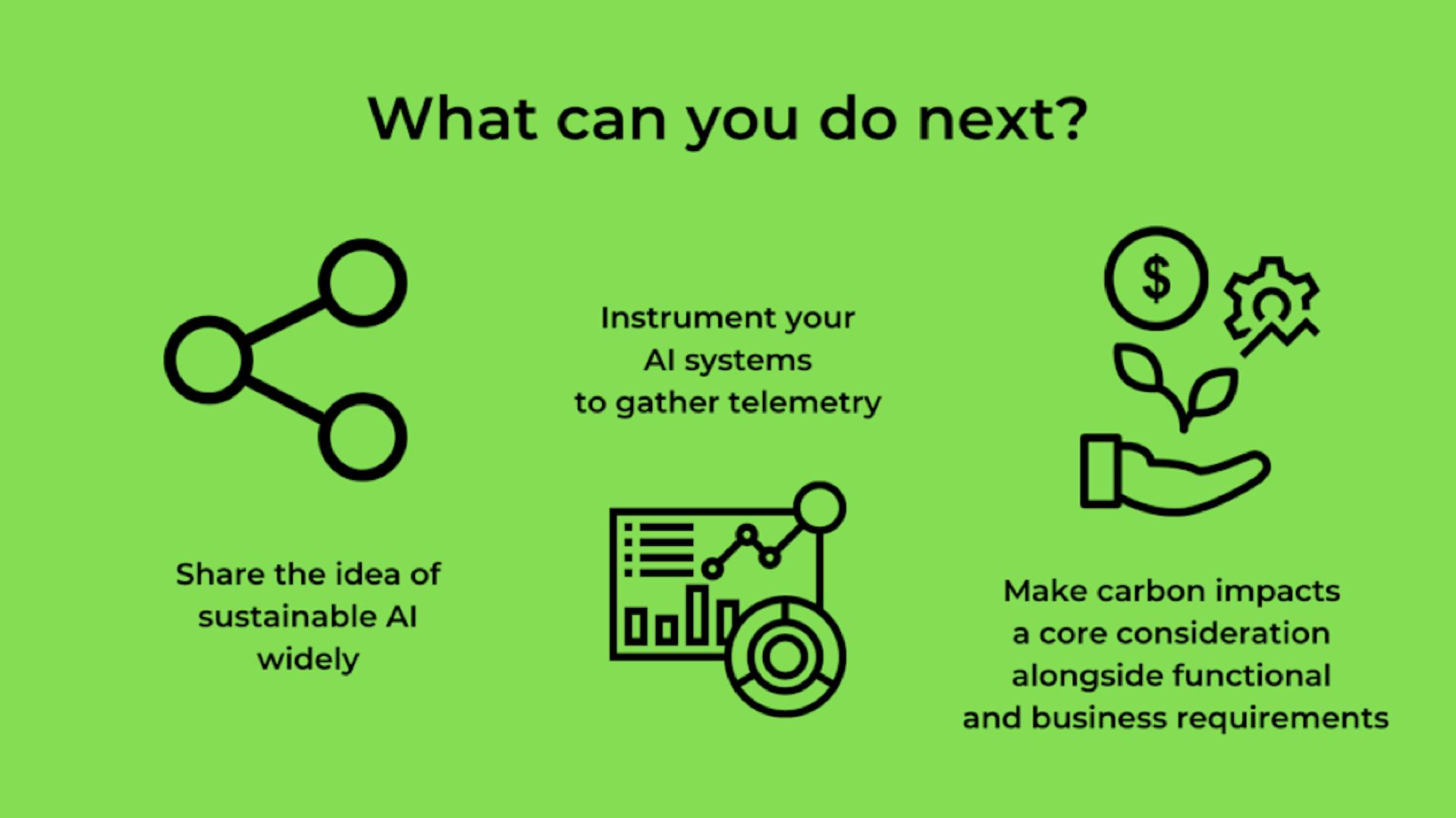
Our first step should be to share the idea of sustainable AI within our research and practice communities. We can make it a standard practice to measure and report the carbon impact of AI systems, encouraging the adoption of "Green AI." Industry leaders can support these efforts, enabling experimentation and fostering a more sustainable AI approach.
Instrumenting AI systems to collect and report energy consumption data is the next step on the sustainable AI journey. Tools like CodeCarbon, carbontracker, experiment-impact-tracker, and scaphandre offer ways to start measuring and managing carbon emissions. Researchers can promote these tools in their labs, integrate them into lab practices, and use them to onboard new members.
Organizations in the AI industry need to make an active choice to prioritize carbon impacts alongside functional and business imperatives. According to Gupta, this is a choice for ethics and strategy. Informed consumers increasingly favor environmentally friendly products and services, directly affecting businesses. Implementing greener AI systems will yield cost savings, bolstering the business case. Even when immediate financial benefits are unclear, committing to sustainability secures a competitive market position and aligns with ESG efforts. While standardized environmental reporting for software and AI is lacking, organizations like the Green Software Foundation are working towards creating interoperable and actionable approaches to inform consumers and guide regulatory efforts. Taking recommended actions at research, practitioner, and management levels will help organizations future-proof and move ahead of the pack.
Join us on October 5 for the virtual GSF event: Responsible AI: Tackling AI's Environmental Challenge
This article is licenced under Creative Commons (CC BY 4.0)