The Green AI Committee (GAIC) was established to unify efforts in innovation and sustainability. Its mission is to guide the Green Software Foundation’s (GSF) strategy in developing knowledge, standards, and tools that minimize the environmental impact of Artificial Intelligence (AI) systems, supporting net-zero goals.
Over a series of workshops at the end of 2024, the GAIC members discussed and agreed on a definition of Green AI. This paper presents the definition as well as a summary of the discussions in that workshop, representing GAIC’s commitment to driving sustainable and scalable AI development while addressing urgent environmental challenges.
The definition below and the following material in this paper were agreed upon by the GAIC by consensus and ratified by the GSF Steering Committee in September 2024.
The Green AI Committee (GAIC) is formed of these members:
Sanjay Podder 🪑 | Accenture |
Thomas Lewis🪑 | Microsoft |
Arun Ravindran | BCG |
Chris Xie | Futurewei |
Federica Sarro | UCL |
Gadhu Sundaram | NTT Data |
Juan José Lopez Murphy | Globant |
Nadim Kapadia | HSBC |
Nisha Menon | Siemens |
Savannah Goodman | |
Vincent Caldeira | IBM/ Red Hat |
Vinjosh Varghese | UBS |
What Is Green AI?
Green AI focuses on reducing the environmental impact of AI systems throughout their lifecycle. It emphasizes the standardization of measurement and metrics to ensure transparency, strengthen confidence in AI technologies, and drive continual improvement.
In their approach to establishing the definition, the committee prioritized the following:
Conciseness: A concise definition enables all stakeholders to grasp the concept of Green AI without being hindered by overly technical or complex language, helping ensure clarity and ease of understanding.
Holistic approach: AI’s environmental impacts are not limited to energy consumption but include resource usage, emissions, and production and disposal of hardware. A comprehensive, system-wide approach is essential to identifying all factors contributing to AI’s footprint. The Green AI Committee is committed to evaluating these impacts across the entire AI lifecycle, ensuring that sustainability strategies address the full scope of environmental challenges and drive effective reductions.
Consideration of the full AI lifecycle: The environmental footprint of AI systems extends beyond operational (inference) and training to include the entire lifecycle, from model development to deployment and end-of-life disposal. Breaking AI down into distinctive lifecycle stages helps identify specific areas for optimization, ensure adequate sustainability efforts at each step, and support environmental accountability across the AI ecosystem.
Focus on the sustainability of AI: The goal of Green AI is to reduce AI’s own environmental impact. Several other initiatives already focus on AI for sustainability, but fewer efforts are dedicated to making AI itself more sustainable. It is essential to keep Green AI’s scope clear and distinct.
What Is Not Green AI?
For the purpose of this paper and in a broader scope of its activities, the Green AI Committee will not consider what AI can do for sustainability, Responsible AI, or AI safety.
While AI itself can be a valuable tool for supporting sustainability initiatives, including these applications would blur the scope of Green AI and risk shifting attention away from the core problem.
Responsible AI focuses on the social and ethical implications of AI systems. It aims to ensure that AI is fair, transparent, unbiased, explainable, and safe, addressing its direct impacts on people and society. While both terms can sometimes overlap, Green AI focuses exclusively on how AI impacts the environment.
Furthermore, AI safety concerns include addressing potential threats such as unintended behaviors in AI systems, a lack of control, security vulnerabilities, and ensuring AI does not cause harm through bias or discrimination. These concerns are related to ethical and social considerations of AI behavior and risk, which fall under the scope of Responsible AI.
Exploring these concepts would broaden the focus beyond the environmental impacts of AI.
Given the evolving nature of AI and its applications, the committee will periodically reassess this scope to ensure it remains aligned with the core objectives of Green AI and GAIC’s mission.
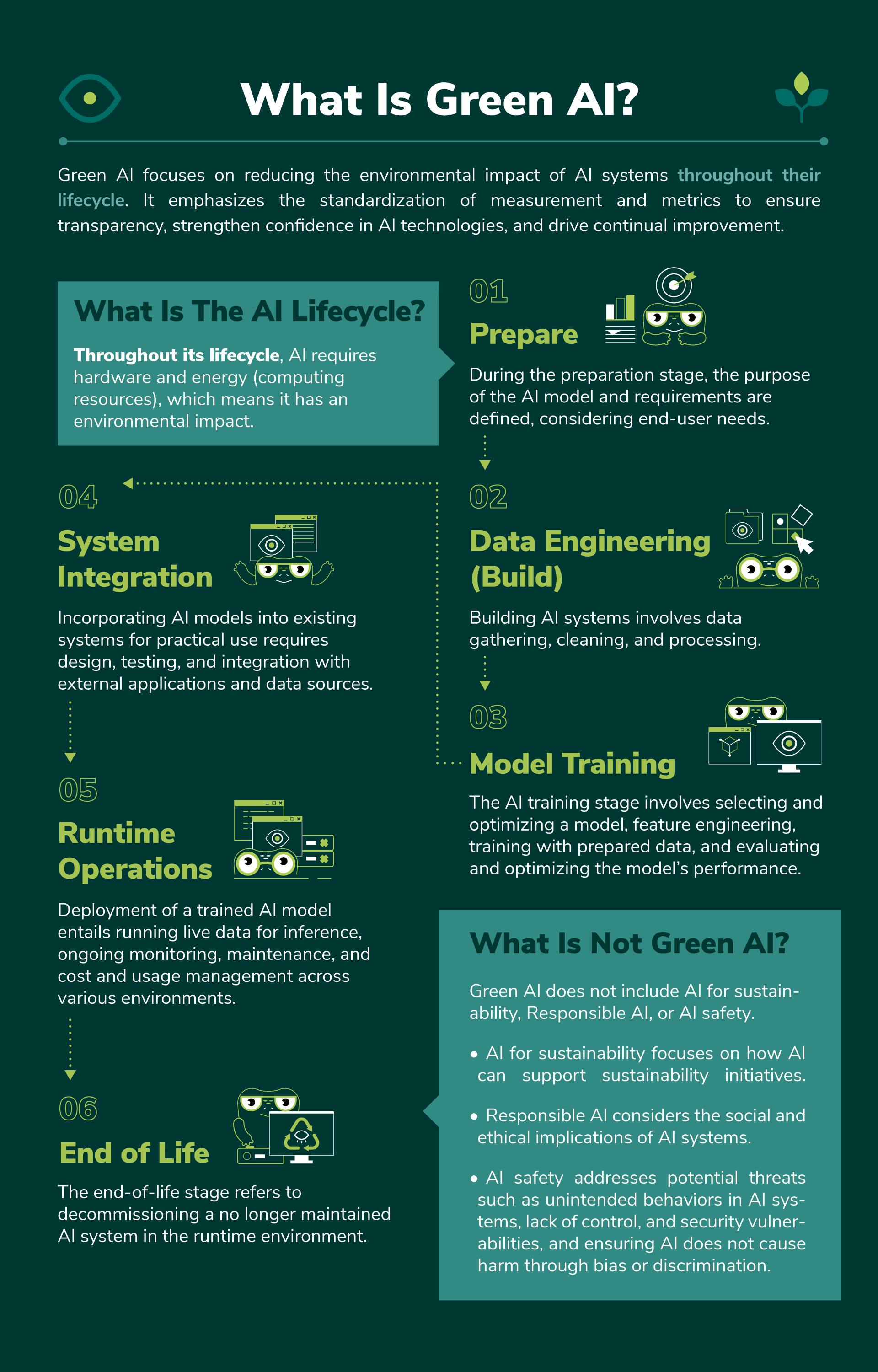
Understanding the AI Lifecycle
The AI lifecycle, as defined by the Green AI Committee, includes the following stages: Prepare, Build (Data Engineering), Model Training, System Integration, Runtime Operations, and Disposal/End-of-Life. Throughout its lifecycle, AI requires hardware and energy (computing resources), which means it has an environmental impact.
Understanding the AI lifecycle and developing standardized methodologies for measuring AI's environmental footprint is critical for advancing sustainability efforts, allowing for a targeted approach at each stage.
Prepare
The AI lifecycle begins with preparation, where the goal is to understand the purpose of the AI model and the problem it will solve. Here, it is also crucial to consider the fundamental question: Do we need AI to solve this problem, or are there alternative, less resource-intensive solutions that can achieve the same outcome? The problem could be anything from recognizing objects in photos to predicting the weather or diagnosing diseases. At this stage, the involvement with end-users is required to understand their needs and expectations. Additionally, clear objectives must be set regarding performance benchmarks, data requirements, response times, and computational constraints. Establishing these is critical to avoid overuse of resource-intensive models.
Data Engineering
Once the objectives and requirements are established, the next step is to build an AI system. As AI needs data to learn, building it will start with gathering data from different sources, such as websites, databases, or human input. Collected data goes through pre-processing, which involves cleaning (checking for potential errors, missing values, and inconsistencies) and normalizing (ensuring the data is in a consistent format for AI to understand). In some cases, synthetic data is created. Synthetic data, generated algorithmically, mimics the patterns and characteristics of real data. It can be used during the training stage as a substitute for test data sets of production or operational data to validate and train models. This allows for cost-efficient and scalable model training, reducing the need for excessive data collection and mitigating potential ethical concerns related to sensitive or private information.
Model Training
With the prepared data, AI enters the training stage, where it learns to recognize patterns and make predictions. Training AI refers to teaching the system how to find connections in data. During training, the appropriate model is selected based on the task characteristics and available data, and optimized to improve its performance. A key part of the training stage, called feature engineering, is the process of transforming raw data into relevant information for use by AI models. Training involves exposing the model to the prepared data to develop and improve its ability to make predictions and decisions using the data provided.
For the training to be successful, several factors must be considered. First, it is essential to determine the right metrics or evaluation measures to track the AI's performance. These might include various measures, such as accuracy (how often the model correctly identifies objects), precision (how many of the identified objects are correct), and recall (how many actual objects are correctly identified).
Model benchmarking allows for the quantitative comparison of different AI models to determine which one is best suited for the task. Evaluating various models can be based on factors such as accuracy, speed, and resource consumption. Training AI models often requires vast amounts of computational resources, especially for large datasets. Setting up distributed training pipelines allows for the division of workloads across multiple devices; it enables faster training and efficient scaling, but also requires careful resource management. Scheduling and resource allocation are key steps in planning when and how to efficiently use resources (like computing power, storage, and memory).
The trained model is evaluated on test datasets to assess its performance on new, unseen data. Model evaluation assesses the accuracy of the performance based on predefined metrics. This is when the model can be iterated to improve efficiency or accuracy. Comparing evaluation metrics across multiple models helps decide which model to deploy.
The following step involves a cost and resource analysis. Understanding how much energy and hardware are used during development and deployment helps determine where to optimize and make improvements. Optimization means minimizing the amount of computational resources required to train and run the AI system without compromising its performance. This could involve simplifying the model, using more efficient algorithms, reducing data redundancy, and deleting or reallocating any underutilized resources.
System Integration
The next step is system integration, which ensures that the AI model works seamlessly with other components, technologies, or systems and can be used effectively in practical scenarios. This could involve integrating AI into a web application, an autonomous vehicle, a smart home system, or any other element that will interact with the AI to complete tasks.
Successful integration requires careful design. Developers determine the architecture, components, and interactions between the AI model and other parts of the system. The design phase ensures that the AI model fits into a larger system, considering factors like performance, scalability, and user experience. The actual integration phase involves integrating an AI model into existing software systems, infrastructure, or workflows through actions such as connecting AI models with external applications, ensuring that they have access to real-time data, implementing authentication, or ensuring compliance with data privacy regulations.
Once the system has been integrated, it must be tested for performance issues or integration errors. Successful system integration testing confirms that the AI model is ready for deployment and can operate in real-world scenarios.
Runtime Operations
Deployment means setting up a trained model for inference, which involves running live data through it. As the model is deployed, it actively runs and performs tasks, including inference, in its deployed environment.
By inference, we understand the ability of a trained AI model to recognize patterns and draw conclusions from new data that it has not seen before.
The AI model that has been deployed will be monitored, evaluated, and maintained. This ongoing process involves tracking the model’s performance, latency, and computational resource usage over time, managing necessary adjustments and updates, retraining it with new data to increase adaptability, and evaluating its behavior in real-time production environments. A stable system ensures that user requests are processed efficiently and in real time. Green AI best practices, such as Green Software Patterns, can provide guidance to users on how to adopt more sustainable practices, such as batching prompts, when interacting with the model to help reduce energy consumption and improve overall system efficiency.
Introducing FinOps practices at this stage helps manage costs and usage related to the adoption of AI models, ensuring sustainable and efficient use. This involves understanding the key components of the FinOps framework and applying them to specific AI environments.
The deployment of AI models spans across consumer (edge) devices, data centers, and cloud environments, with each having its own monitoring requirements due to unique capabilities and limitations.
End-of-Life
The end-of-life stage refers to decommissioning a no longer maintained AI system in a runtime environment.
The AI Lifecycle: Key Stages and Elements
Prepare | Research and Problem Definition: - Define the problem and identify solution requirements (engaging with end-users) - Identify the problem + outcome - Stakeholder engagement & expectations |
Data Engineering (Build) | Data Identification and Collection: - Data Collection - Collect data sources and prepare them (cleaning, normalizing) Data Preparation: - Data Pre-Processing - Synthetic data generation |
System Integration | Model Development: - Identify appropriate models and prepare them - Feature Engineering Model Training: - Identify appropriate evaluation measures - Model Selection Benchmarking - Set up distributed training pipelines - Define scheduling and resource allocation Model Testing Model Evaluation: - Cost /Resource Analysis - Optimize compute and resource cost spend System Integration: - System Integration Design - System Integration Development - System Integration Testing |
Runtime Operations | Model Deployment: - Deployment, roll-out, and people training. Model Operation (including inferencing): - Inferencing Monitoring: - Monitoring, iteration & reporting - Model Performance Monitoring - FinOps - Consumer (Edge) Device / Datacenter / Cloud Usage Maintenance (for example, model degradation) |
End-of-Life/ Disposal | Decommissioning an AI system |
Why Is Green AI Important?
In the last decade, AI has seen accelerated progress in a range of capacities, yet this advancement has increased its environmental impact and put a strain on energy systems. The development and deployment of AI models are responsible for adverse environmental impacts, including growing carbon emissions, water usage, and energy consumption. Some predictions expect data centre emissions to rise from 180 Mt to 300 Mt in the Base Case by 2035, making them one of the fastest-growing sources of emissions.
The rapid and divergent nature of AI development has led to growing concerns and controversies around balancing its potential benefits and negative environmental consequences.
While the challenges are significant, the GAIC believes a more sustainable future for AI is achievable. It is crucial to underline that Green AI is not a standalone solution but a fundamental pillar of a broader sustainability ecosystem. By adopting Green AI practices alongside collaboration, policy innovation, and technological advances, we can align AI’s growth with planetary boundaries.
Addressing Key Challenges in Green AI: Priority Projects
Despite rapid advancements in AI, practitioners and users currently lack clear guidance and knowledge on how to measure, reduce, and report AI impacts. This absence limits public awareness and hinders efforts to address AI’s environmental footprint, making it more challenging to develop AI sustainably.
To support further sustainable development, deployment, and use of AI systems, the committee will pursue the following initiatives:
Green AI for Practitioners Course (also referred to as Green AI White Paper)
The Green AI White Paper will be produced in collaboration with GSF members to increase awareness of Green AI and understanding of the implications of AI development. It will explain the fundamental principles of Green AI, developments, and solutions, and provide practical, actionable recommendations for practitioners, including guidelines for measurement.
The project is led by Thomas Lewis (Microsoft).
SCI for AI Standard
In collaboration with the GSF Standards Working Group, the SCI (Software Carbon Intensity) specification will be extended to cover AI and address the challenges of measuring AI carbon emissions through the AI lifecycle. The purpose of the proposed specification is to support AI practitioners and decision-makers in understanding and reducing the carbon footprint of AI systems by providing a reliable, consistent, and comparable measure.
It is crucial to highlight that energy efficiency does not always equate to carbon efficiency. This is due to several variables, including regional differences in electricity carbon intensity, hardware configurations, and timing of deployment, all of which can significantly affect overall environmental impact. Therefore, the integration of real-time carbon intensity metrics is essential to achieving truly sustainable AI operations.
As part of this initiative, the committee will focus on decreasing barriers to public awareness of the carbon intensity of AI.
The project is led by Navveen Balani (Accenture).
Furthermore, the committee will closely monitor and actively respond to the evolving policy landscape (for example, the EU AI Act and Singapore IMDA’s Digital Sustainability Guidelines). This will aim to support clarity, build trust, and encourage a collaborative approach in policy-making.
To support real-world adoption, the committee will work closely with the GSF to promote and integrate open-source observability tools into standard development and deployment practices. These tools can help practitioners monitor energy use, track carbon intensity in real time, and make informed decisions to reduce environmental impact.
By championing open tools, the committee aims to ensure that sustainability practices are accessible, transparent, and scalable across different environments and organizations.
Get Involved
Collaborate: The Green AI Committee recognizes that addressing AI’s environmental impact requires broad collaboration between industry, academia, and policymakers to establish comprehensive standards that prioritize transparency, software and hardware efficiency, and environmental accountability.
We encourage AI hardware organizations to actively participate in this conversation. Involving a broad spectrum of AI and ICT organizations will help drive consensus on best practices, optimize energy efficiency in model training and deployment, and ensure that sustainability is a fundamental design principle rather than an afterthought.
Become a member: To actively participate in the work of the Green AI Committee and shape standards and policy in green software, join us. To ensure clarity, a Steering Member status is typically required for a guaranteed seat on the committee, while standard members may be considered through a nomination process. We’re committed to making this process open and straightforward for all who wish to contribute.
Further reading
https://wedocs.unep.org/handle/20.500.11822/46288;jsessionid=81D6D7C73D7BA6E37CF4AC150AE8849B
https://news.mit.edu/2025/explained-generative-ai-environmental-impact-0117
https://www.computer.org/csdl/magazine/so/2024/05/10629208/1Zdj4SWzTIQ
This article is licenced under Creative Commons (CC BY 4.0)